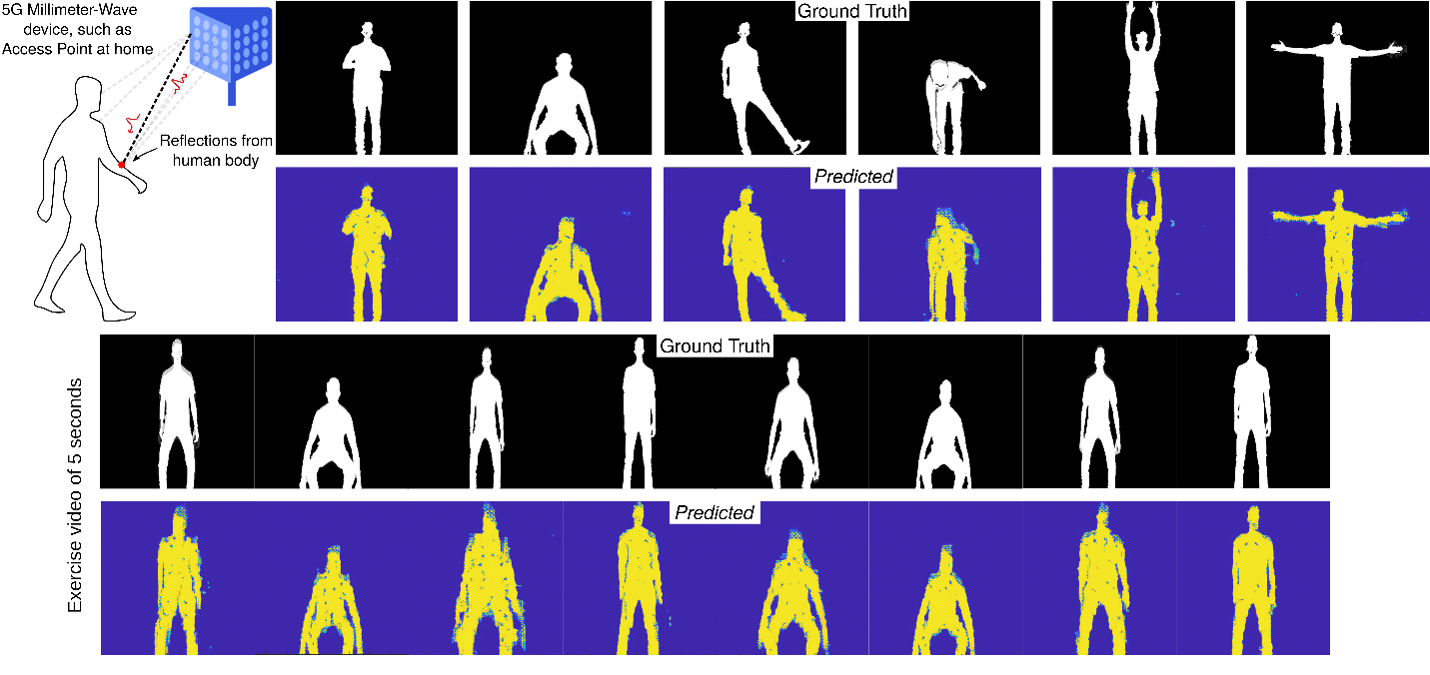
The need for understanding and perceiving at-home human activities and biomarkers is critical in numerous applications, such as monitoring the behavior of elderly patients in assisted living conditions, detecting falls, tracking the progression of degenerative diseases, such as Parkinson’s, or monitoring recovery of patients’ during post-surgery or post-stroke. Traditionally, optical cameras, IRs, LiDARs, etc., have been used to build such applications, but they depend on light or thermal energy radiating from the human body. So, they do not perform well in occlusion, low light, and dark conditions. More importantly, cameras impose a major privacy concern and are often undesirable for users to install inside their homes.
Now a team of researchers from the Systems Research on X laboratory at the University of South Carolina has designed a monitoring system, called MiShape, based on millimeter-wave (mmWave) wireless technology in 5G-and-beyond devices to track humans beyond-line-of-sight, see through obstructions, and monitor gait, posture, and sedentary behaviors. This system provides an advantage over camera-based solutions since it works even under no light conditions and preserves users’ privacy. By processing mmWave signals and combining them with custom-designed conditional Generative Adversarial Networks (GAN) model, they demonstrated that MiShape generates high-resolution silhouettes and accurate poses of human body on par with existing vision-based systems.
The findings are reported recently in the ACM Journal on Interactive, Mobile, Wearable and Ubiquitous Technologies (IMWUT) in a paper co-authored by UofSC graduate students, Aakriti Adhikari and Hem Regmi, and UofSC faculties of computer science and engineering department, Dr. Sanjib Sur and Dr. Srihari Nelakuditi. It was also recently presented at the highly selective international conference, ACM UbiComp 2022, by Aakriti Adhikari.
In their proposed approach, they first train a deep learning model based human silhouette generator model using mmWave reflection signals from a diverse set of volunteers performing different human poses, activities, etc., and then run the model to predict the silhouette of unknown subjects performing unknown poses, which are not part of the training process. The silhouette can then be used to generate a body skeleton, which can be tracked continuously, even under obstructions or low-light, for monitoring human activities automatically. Furthermore, the system can generalize to different subjects with little to no fine-tuning.
This research is an example of an emerging paradigm called Sensing for Well-being. It enables ubiquitous sensing techniques so that devices and objects become “truly smart” by understanding and interpreting the ambient conditions and activities with high precision, without relying on traditional vision sensors. “Through experimental observations and deep learning models, we extract intelligence from wireless signals, which, in turn, enable ubiquitous sensing modalities for various human activities and silhouette generation,” says Prof. Sur. The authors are also collaborating with researchers from the Arnold School of Public Health and doctors from the School of Medicine to bring these technologies to practice. Another application of this work is in monitoring human sleep quality and postures with ubiquitous networking devices, such as next-generation wireless routers at home. “We can use mmWave wireless signals to automatically classify, recognize, and log information about sleep posture throughout the night, which can provide insights to medical professionals and individuals in improving sleep quality and preventing negative health outcomes,” Sur says.
The research was supported by the National Science Foundation, under the grants CNS-1910853, MRI-2018966, and CAREER-2144505, and by the UofSC ASPIRE II award.